Kanvas Bio's State of the Art AI Models & Reflections from JPM 2024
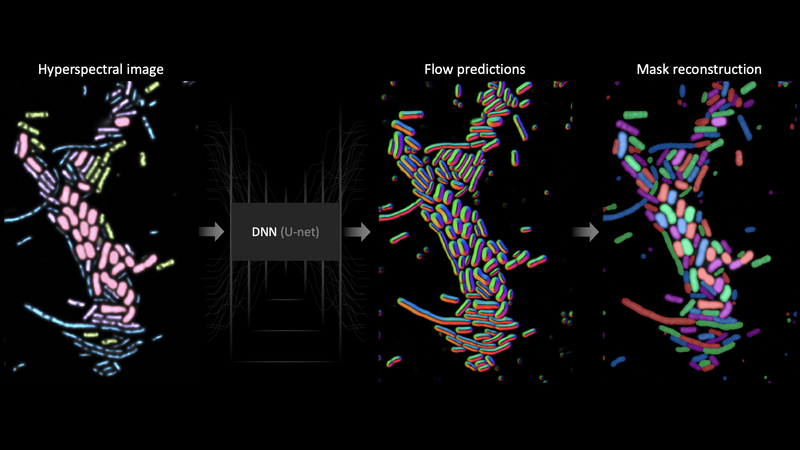
AI Use Cases are Broadening: Key Takeaways from the 2024 J.P. Morgan Healthcare Conference and a Deep Dive into Kanvas Bio’s State of the Art Models
Posted by Kanvas Bio
We had the privilege of attending the J.P. Morgan Healthcare Conference earlier this month, which brings together thousands of global healthcare leaders, emerging companies and investors. Given the scale and caliber of the event, many major media outlets published in-depth recaps; however, as an early-stage startup operating in the trenches of the health tech sector, we wanted to chime in with our own takeaways:
One of the most common topics of conversation was how healthcare M&A will increase in 2024. Large pharmaceutical companies will need to fill their late-stage pipelines to offset patent expiries and potential further pricing pressure from the Inflation Reduction Act. For startups, raising capital will remain an issue until interest rates lower and/or VCs achieve more exits through M&As or IPOs.
Everyone we spoke to said they’re using AI, but that’s no longer good enough. The consensus was, companies need to be able to explain what they’re using AI for, and they need to be able to defend if their models are high-quality, if those models are trained on high-quality data, and how they’re solving for AI bias and hallucination.
There was palpable buzz around cell and gene therapy. We’ll be especially interested to see if the live biotherapeutic product (LBP) space can benefit from the path human cell therapies are currently forging.
Unsurprisingly, AI was a hot topic and we were excited to witness a broadening number of AI use cases that extend across the R&D value chain (clinical trial recruitment, protocol writing, study design and predicting function from structure). We also had several conversations about the tradeoffs between training as a generalist tool (like ChatGPT) with potential hallucinations vs. training for very specific tasks with no risk of hallucinations.
Given the focus on AI at the conference, we wanted to address how Kanvas Bio is using AI, and share a first hand look into how we’re training our models and the challenges we’ve had to overcome:
Kanvas Bio is using AI for processing and interpreting hyperspectral image data at the single-cell level. More specifically, we’re using our own proprietary AI models to decode the taxonomy of microbes and identity of host cells from high-dimensional spectral images of biological samples, such as tissues and fecal matter. This hypothesis-free framework allows us to build microbe-microbe and host-microbe interaction maps, and we’re using these maps to create large atlases of host-microbiome interactions. These high quality atlases will be used to generate hypotheses to understand the mechanisms of Kanvas Bio’s novel therapeutics.
Our AI models and training requirements are unique, due to the novel nature and massive amounts of data we’re working with. But at Kanvas Bio, we have the advantage of using spectral fluorescent images to improve our training data. We’re leveraging this high-dimensional spectral data to generate high-quality annotations of complex microbial environments, which would otherwise be challenging with traditional non-spectral fluorescent images.
Because Kanvas Bio is leading the field of mapping host-microbiome interactions, often we’re generating data that’s never been seen before – and that presents challenges. For example, we had to adapt our analysis strategy for tissue samples to address problems such as high density of microbes that are often stacked on each other, presence of bright food particles, and background autofluorescence. All of these challenges require careful annotation of ground truth data for training an AI model, which is an active area of development.
To successfully leverage complex data that’s never been seen before, we use Omnipose, a deep-learning-based image segmentation platform created by one of our team members. Omnipose uses a deep neural network architecture called a U‑net to transform images into vector field components that define cell boundaries and cell interiors. This approach works well on complex tissue images not only because a U‑net can be trained on any image type, but also because the network of Omnipose specifically is trained to predict a vector field that is universally applicable to any cell morphology. In other words, the U‑net can be trained to transform any image type, and its transformations can describe any cell type.
Because our biggest challenge is the curation of ground-truth training data, we plan to use state of the art image synthesis, such as diffusion models, in combination with optical physics simulations to generate synthetic training data for image segmentation and classification. This approach of synthesizing labels and corresponding images will allow us to build annotated datasets much larger than those we could create manually, which can be used to build models that are vastly more capable and generalizable.
Looking ahead, we’re especially excited to leverage the unprecedented host-microbiome insights that we’re generating by building AI drug discovery models trained on our proprietary data to understand the design principles for clinically beneficial microbial communities. Together, these principles set the stage for designer precision microbiome therapeutics that go well beyond what can be achieved with fecal microbiota transplants (FMTs) and conventional LBPs. The potent combination of our proprietary strain libraries and cutting edge AI models will pave the way for the creation of “supercharged” designer microbiomes, where key functional and metabolic capabilities are precisely added to existing microflora. Ultimately, Kanvas Bio is on a mission to produce precision microbiome therapeutics that exceed the limitations of fecal microbiota transplantation (FMT) and conventional live bacterial products (LBPs), and continually refining our AI models is a key component of achieving that mission.
To learn more about how Kanvas Bio is using AI to accelerate the discovery and development of microbiome-based therapeutics, reach out to us directly!